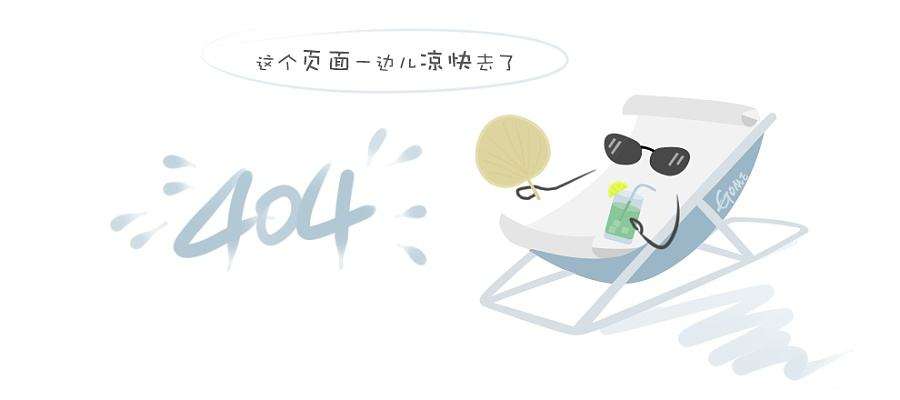
date: semptember 16, 2021
time: 16:30 pm
venue: room 0411, teaching building 0#, jiuli campus
event details:
lecturer: associate professor feng ma
about the lecturer:
feng ma, associate professor, phd supervisor, is currently a teacher in the department of finance. now he presides over one project from the national natural science foundation of china, one project from the ministry of youth and humanities and social sciences of the ministry of education, and participates in a number of national-level projects. he has published more than 80 academic journals, and his main research work has been published in journal of banking & finance, journal of empirical finance, international journal of forecasting, journal of forecasting and other journals. in april 2021, he was selected as the 2020 elsevier china highly cited scholar (applied economics).
about the lecture:
this study develops a prevailing shrinkage method, lasso with a markov regime-switching model (mrs-lasso), to predict us stock market volatility. in total, 17 well-known macroeconomic and financial factors are used in this research. the out-of-sample results reveal that the mrs-lasso model can successfully predict volatility from statistical and economic viewpoints. we further investigate the predictability of mrs-lasso in terms of the different market conditions, business cycles, and variable selection. three factors (equity market returns, short-term reversal factor, and consumer sentiment index) are the most frequent predictors. to investigate the practical implications, we construct the expected variance risk premium (vrp) by using volatility forecasts generated from the lasso and mrs-lasso models to forecast future stock returns and find that those models are also powerful.